Transformative Solutions for Asset Heavy Industries
From AI-driven insights to customized applications, we deliver results for the most demanding operations.
Collaborative & Efficient
We blend deep industry expertise, advanced data science, and agile software engineering to deliver impactful AI solutions. With backgrounds in industrial engineering and consulting, we take a top-down approach to solving complex problems, working closely with customers to ensure solutions are valuable, scalable, and user-friendly
Our data scientists prototype quickly using first-principle physics and advanced analytics. Our software engineers leverage the Arundo Foundation to build lightweight, fast, and maintainable applications. Together, we deliver results with speed and precision.
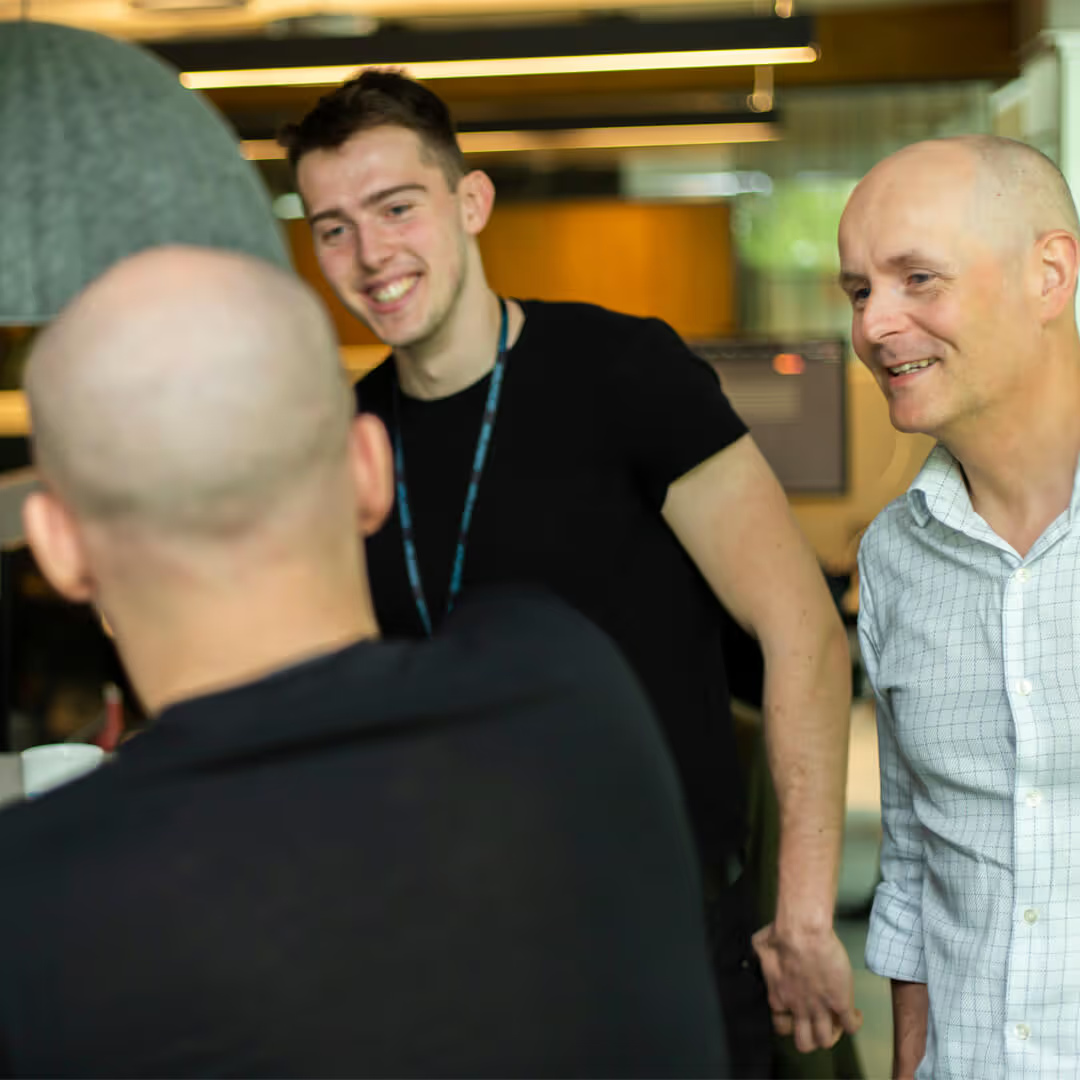
A recipe to get started
Connect your data
Connect your data to the Arundo AI Foundation industrial data store.
Create your domain
Describe your assets and their interconnections in AI Foundation Studio. Create computes and connect machine learning models.
Generate Insights
Let Arundo AI Foundation’s scalable model orchestration generate insights.
Consume & Learn
Converse with Arundo AI Companion to get new insights from the data in your domain - or build your own tailored AI application using our APIs.
Expand at your pace
Start small and focus on high-value use cases. Build out your domain in Arundo AI Foundation as you go.
Let’s connect the dots!
Join other leading industrial companies and discover how Arundo’s AI Foundation adds insight and intelligence to your operations